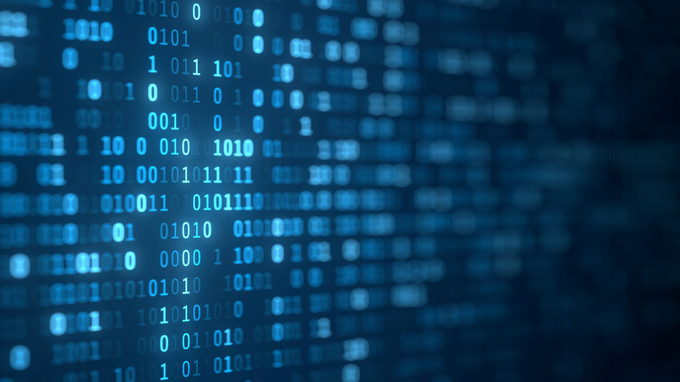
Computer Science
Welcome to the Department of Computer Science
The Department of Computer Science celebrated its 50th birthday in 2018. Its first students graduated as the University of Saskatchewan Regina Campus became the University of Regina. Our programs have attracted faculty members and students from all over the world. The first Department Head, Lawrence Symes, was an alum of the Regina Campus. Grace Hopper visited the campus in 1972. The Computer Science B.Sc. Honours Program was accredited by CIPSAC in 1983. The Department is the longest continuously accredited in Canada. An M.Sc. program was started in 1981 and a Ph.D. program was started in 1992.
Computer Science is the study and application of computing principles and techniques. With foundations in theory, methods, and programming, computer scientists develop software solutions to a broad range of real-world problems. Computer Science encompasses a well-established set of sub-disciplines that include information systems, artificial intelligence, systems and security, human-computer interaction, and data science. The dynamic nature of Computer Science means that these sub-disciplines are in a constant state of development refinement, creating potential for the emergence of new sub-disciplines such as quantum computing and ambient intelligence. Computer Science is inherently interdisciplinary, drawing upon and contributing to a broad range of academic pursuits.
Students may pursue full-time or part-time study leading to a Diploma or a Degree (BSc, MSc, or PhD). A co-operative education designation (through the Centre for Experiential and Service Learning), which allows paid work experience alongside studies, is available with BSc and MSc programs.
Contact the Department of Computer Science
Our Programs
Explore Computer Science
Upcoming Events
Our Purpose
Mission
- To enable research excellence through the development and enhancement of research clusters and the leveraging of local, national, and international collaborations.
- To provide students with a robust computer science education that focuses on knowledge and skill development, experiential learning, and opportunities to envision and create novel Computer Science solutions to real-world problems.
- To align the size of our department with the importance of computer science in the modern world and the demand for our research ability and academic programs.
Vision
To pursue and share innovative and impactful research, to deliver high-quality undergraduate and graduate programs of study, and to be ranked amongst the top 300 Computer Science departments in the world (according to Times Higher Education)
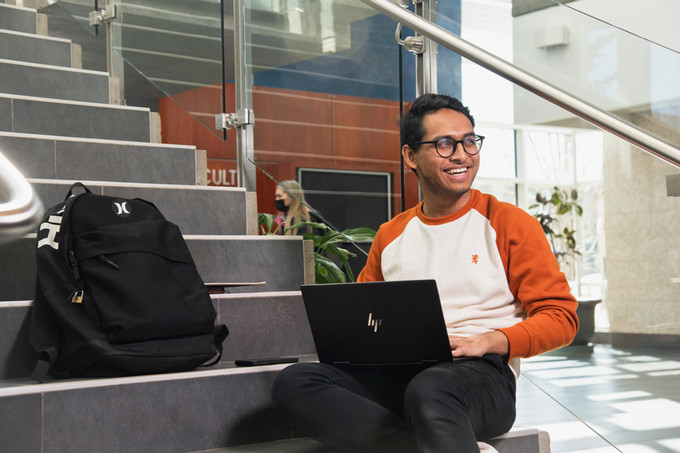