U of R Stories
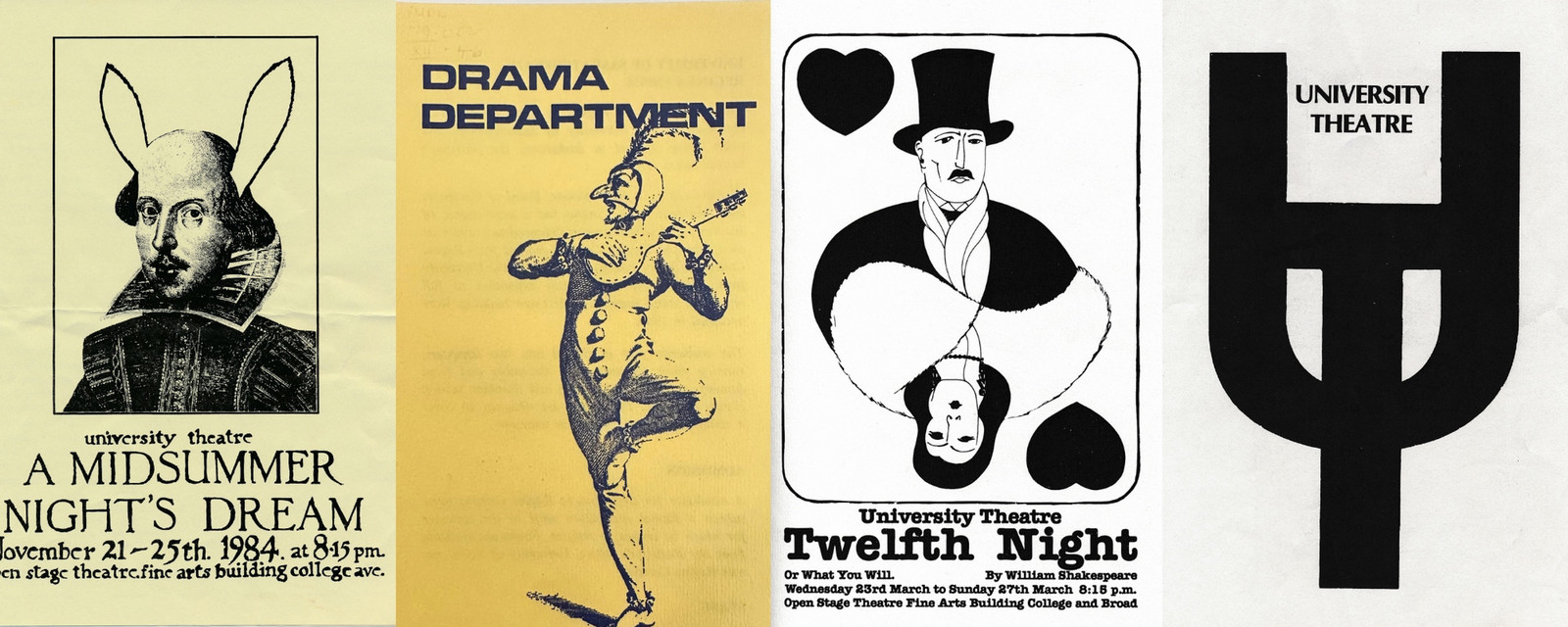
Cue the Next Act: 50 Years of Theatre at the University of Regina
Recent Posts
Planning a Trip? Imagine an App that Makes it Easier by Connecting You with Local Experts
A U of R graduate of the Human-Centred Computing program used empathy-driven design to develop a prototype for a new travel app.
Cue the Next Act: 50 Years of Theatre at the University of Regina
Celebrating 50 years of theatre, creativity, and bold reinvention from the U of R’s Theatre Department
U of R Celebrates the 2025 Queen City Pride Parade
Catch the U of R in action at this year’s Queen City Pride Parade.
Cadmus Delorme Named Next Chancellor of the University of Regina
On July 1, 2025, Cadmus Delorme will take over from Pam Klein to become tenth chancellor of the University of Regina.
Longtime U of R Photographer, Don Hall, Awarded Honorary Degree At 2025 Spring Convocation
The photographer captured campus life with a rare blend of technical skill, artistic vision, and heartfelt emotion.
The Honourable Dr. Lillian Eva Quan Dyck, O.C. Awarded Honorary Degree
Pioneering neuroscientist, former Canadian senator, and advocate for Indigenous and women's rights receives U of R’s highest honour.
Longtime U of R Advocate, Dr. Jim Tomkins, Awarded Honorary Degree At 2025 Spring Convocation
Former president, past chancellor, and lifelong University supporter awarded U of R’s highest honour, an honorary degree, at the 2025 Spring...
Congratulations! U of R Celebrates the Graduation of More Than 2,400 Students
Spring 2025 Convocation marks academic milestone with four ceremonies over three days.
The Honourable Russ Mirasty S.O.M., M.S.M., awarded Honorary Degree at Spring Convocation
First Indigenous Lieutenant Governor of Saskatchewan receives U of R’s highest honour.